Sie befinden sich hier: Home › Kreditwesen › Themenschwerpunkte › Aufsätze › Consumer and asset prices - some recent evidence
Aufsätze
15.06.2015
Consumer and asset prices - some recent evidence
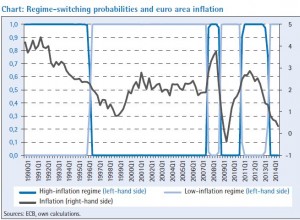
Chart: Regime-switching probabilities and euro area inflation Sources: ECB, own calculations.
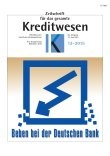
Dieser Artikel ist Teil unseres Online-Abo Angebots.